We want to make, with the use of BDA, more transparent people‘s interactions and communications in organisations. What are their demands for learning in these situations? The risk is that an insensitive use of BDAmay overwhelm people and organisations. Management of big data needs awareness of our cognitive, ethical and organisational capabilities. For instance, with reference to the justice system, it is dangerous to have judges sentencing individuals with the support of big data, based on appreciating their current and possibly their future behaviour, without the monitoring of these individuals‘ contextualised situations, that is, without efforts to improve organisational processes. Also, in commercial activities, it may be ineffective for companies to use marketing algorithms that ignore individual and structural competencies. Overwhelming people with marketing data, abusing their privacy, is a recipe to alienate them. To use BDA to understand and predict individuals‘ insights in their organisations is necessary. Many, among them (Bello-Orgaz, Jung, & Camacho, 2016)use social media data analysis methodology for that purpose. (Perko, 2017) analyses the behaviour of finance officers to predict their future actions. (Bellomo, Clarke, Gibelli, Townsend, & Vreugdenhil, 2016) explore behaviour in evacuation crowd dynamics to prevent or mange critical situations.
2.1.1 The Viable System Model and Viplan Methodology. The Viable System Model (Beer, 1979, 1981, 1985) – VSM- and the VIPLAN Methodology (Espejo, 1993; Espejo & Reyes, 2011) have been used to discuss the braiding of organisational learning (Espejo, et al., 1996) and technological processes. Model and methodology are supported by a systemic epistemology, which highlights holism, in particular the relevance of communications, interactions and complexity in organisations.
In this paper we adapt theViplan Methodology to analyse BDA in organisational system (Espejo & Reyes, 2011). This adaptation is presented in figure 2. It brings together data management and organisational learning. This is a methodology for organisational and individual learning and for data management. It is driven by two learning loops; the first relates to the issues we want to make transparent in an organisation and callthe issue learning loop (the white loop) and the second is an organisational learning loop (the black loop). Observing, assessing, designing and implementing drive both loops. For issues of concern, such as those we referred above (e.g. sentencing in the justice system, adverting in enterprises, financial reporting and so forth) we refer to, observing issues of concern with the support of big data; assessing these observations from the perspective of actors and agents interactions and the variety operators dealing with their complexity asymmetries; designing improved variety operators to make interactions more effective and finally, closing the loop, implementing new variety operators which generate new data. However, this issue-learning loop happens in the context of an organisational system that is learning as well. This learning aims at improving this context by assessing the organisation structure embedding the issue of concern, designing structural improvements and implementing these improvements. This organisational learning is necessary to deal with ethical and performance issues relevant to the issues of concern.
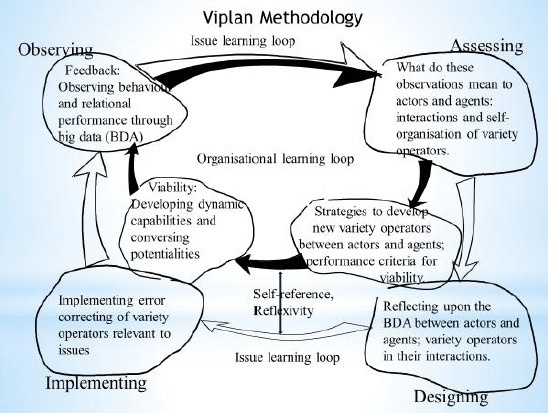
Figure 2. The Viplan methodology (adaptation of Espejo, 1993)
3. The learning model related hypothesis
3.1 BDA redefines the data sharing, objectives insights and knowledge concepts. Controlled sharing of data, objectives insights and knowledge between peers, sub-systems, organisation – even the competing ones, elevates the understanding of the whole system, and provides a higher viability probability comparing to the others. (Perko, Primec, & Horvat, 2015) for instance elaborate the business, law and ethical perspectives of sharing business partners‘ behaviour data.
3.2 The generation and use of tacit knowledge is redefined:
• Thenumber of issues analysed is dramatically increased: from maybe hundreds, analysed by an individual, to millions, analysed with the wisdom of the crowds.
• Theamount of data analysed per issue is increased, compared to standard statistical analysis, even when experts suggest that the variety of data they analyse in a situation, cannot be analysed with an algorithm.
• Theissue analysis can clearly explain the reasoning behind the outcome prediction, the risk of ignoring important attributes is reduced.
• The prescriptive analytics can support or even replace, within limits, the expert decision taking. In the decision situations, where the Big Data based reasoning capacity matches the complexity of the environmental situations. A clear example of such development are the traffic/ routing/ logistics management systems, where drivers are relying on the navigation systems.
3.3 The generation and use of theoretic knowledge is redefined:
• The theoretic knowledge generation can become data driven. Instead of confronting tacit knowledge of multiple experts, the models of behaviour can be extrapolated directly from the data of the issues themselves. Standardisation and the relevance of the issues would no longer be matter of expert perception, but data based. An example: in financial institutions operational risk management directives are based on the risk probability and consequences perception of the risk management experts. Based on the experiences in the financial sector, it is easy to conclude, that they often put emphasis on the wrong risks.
• Storing the theoretic knowledge: the big data theoretic knowledge is stored in the form of predictive or prescriptive models or is based upon reports, produced by the models. It upgrades and complements the existing theoretic body of knowledge.
• The theoretic knowledge use is instance based. The experience, stored in models is applied upon the instance data. The elaborations, predictions and prescriptions are used to support decision taking, communication and automation. The main advantage of using big data based models is, that users do not need to analyse the complete theoretic backgrounds, but can focus on instances.
The backdraft of implementing BDA is, that it cannot imply all of the knowledge on complex issues, especially if the data quality or the number of cases recorded is not sufficient to provide reliable models. Therefore Big Data stored knowledge should be focussed on supporting processes, related to relatively simple instances, with multiple repetitions.
3.4 The mechanisms to manage the representation of the complexity are redefined. The main goal of an organisation is to coordinate individual capabilities to achieve itsindividually defined goals. The knowledge of an organisation is a complex combination of structured rules and shared tacit knowledge on multiple levels, gained from previous experiences – gained through previous activities or acquired from the environment.
Figure 3. Learning by observation
BDA tools use a different approach than Business intelligence. Instead of reducing variety and focusing solely on financial business outcomes, they provide the option to understand, predict or even propose activities on the detailed data, provided by the organisation.
3.5 The inter and trans team learning and experience sharing is redefined.